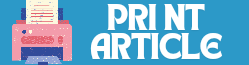
Addressing Data Privacy Concerns in Healthcare AI Applications
In recent years, the Healthcare AI industry has witnessed a transformative shift towards the integration of artificial intelligence (AI) into various aspects of patient care, diagnosis, and administrative operations. AI has shown tremendous potential in improving healthcare outcomes, streamlining workflows, and reducing costs. However, with this innovation comes an inherent challenge: data privacy. The sensitive nature of health data makes privacy concerns particularly critical when AI is deployed in healthcare applications.
Data privacy is a fundamental issue because healthcare data contains personally identifiable information (PII) and protected health information (PHI), both of which are highly regulated under laws like the Health Insurance Portability and Accountability Act (HIPAA) in the U.S. and the General Data Protection Regulation (GDPR) in Europe. Given these privacy regulations, ensuring that AI applications adhere to strict data privacy standards is paramount to fostering trust, protecting individuals’ rights, and ensuring compliance with legal frameworks.
In this article, we will explore the various concerns related to data privacy in healthcare AI applications and how they can be addressed effectively.
1. Data Sensitivity and the Need for Strong Privacy Protections
Healthcare data, by nature, is highly sensitive. This data includes a patient’s medical history, diagnosis, treatment plans, lab results, medication records, and demographic information. The integration of AI into healthcare means that vast amounts of this sensitive data are often processed, analyzed, and stored in digital formats, creating new avenues for potential data breaches, unauthorized access, or misuse.
AI models, particularly those used in predictive analytics, diagnostic tools, and personalized treatment planning, require large datasets to train and improve their performance. However, the more data an AI model has access to, the greater the risk that sensitive information could be exposed or misused. This makes it critical to establish strong data privacy measures throughout the entire lifecycle of healthcare AI systems—from data collection and storage to processing and sharing.
2. Regulatory Compliance: HIPAA, GDPR, and Beyond
Compliance with data privacy laws is a cornerstone of building trustworthy AI applications in healthcare. In the U.S., HIPAA mandates that healthcare organizations and their business associates protect the privacy and security of PHI. HIPAA sets standards for how health information should be accessed, shared, and protected in digital formats, and these standards apply to AI applications that process or analyze health data.
In Europe, the GDPR provides a robust framework for data protection and privacy, which applies to any organization handling personal data of EU residents, including healthcare organizations using AI technologies. The GDPR imposes strict requirements on data controllers and processors, including the need for explicit consent from individuals before their data can be used for processing and analysis. Additionally, the regulation introduces the concept of data anonymization and pseudonymization, which can be useful for ensuring privacy while enabling the development of AI models.
Healthcare organizations and AI developers must implement measures to comply with these laws, ensuring that they maintain strict control over how health data is used, stored, and transmitted. Non-compliance can result in severe penalties and damage to an organization’s reputation.
3. Data Anonymization and De-Identification
One of the most effective ways to protect patient privacy while still enabling the use of data for AI training is through data anonymization or de-identification. By stripping personally identifiable information from datasets, healthcare organizations can reduce the risk of exposure while still providing valuable data for machine learning algorithms.
Anonymization involves removing or obfuscating all direct identifiers, such as names, addresses, and contact details. However, de-identification allows for certain indirect identifiers (e.g., age, gender, zip code) to remain, as long as there is no reasonable way to link this information back to an individual.
Anonymized data is valuable for training AI models while reducing the risk of personal data exposure. This practice not only helps organizations comply with data protection regulations but also mitigates the risk of identity theft, unauthorized access, and other malicious activities.
4. Federated Learning: Decentralizing Data Processing
Federated learning is a relatively new approach that has gained significant attention in the AI and healthcare fields as a means to address privacy concerns. This decentralized machine learning technique allows AI models to be trained across multiple devices or data sources without the need to centralize sensitive data.
With federated learning, healthcare providers and AI developers can build AI models without ever directly accessing the raw patient data. Instead, the data stays securely within its original location (such as a hospital or clinic), and only model updates or parameter changes are shared between participants. This approach minimizes the risk of data breaches and ensures that sensitive health information is never transmitted across networks or stored in centralized locations.
Federated learning is particularly useful in healthcare, where data privacy and security are critical concerns. By reducing the need for centralized data storage, federated learning enables healthcare organizations to leverage the power of AI while maintaining strict control over patient data.
5. Blockchain Technology: Ensuring Transparency and Security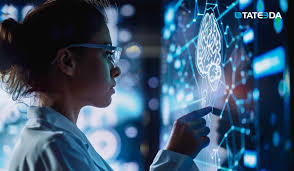
Blockchain technology has emerged as a potential solution for improving data privacy and security in healthcare AI applications. Blockchain offers a decentralized and tamper-proof ledger system, which can be used to record and track every interaction with healthcare data. By using blockchain, healthcare providers can create a transparent, immutable record of how data is accessed, processed, and shared, ensuring that all actions are traceable and auditable.
Blockchain’s distributed nature ensures that sensitive health data is not stored in a single centralized location, making it less vulnerable to attacks. It also allows for encrypted data sharing, ensuring that only authorized parties can access and process patient data.
Additionally, smart contracts built on blockchain can automate privacy protections and consent management, ensuring that AI applications only use data in accordance with patient preferences and legal requirements. Blockchain’s ability to provide a secure, transparent, and immutable record of data transactions makes it a promising technology for addressing privacy concerns in healthcare AI.
6. Ensuring Informed Consent
Obtaining informed consent from patients before using their data for AI applications is a fundamental aspect of data privacy. Informed consent ensures that patients are fully aware of how their data will be used, what kind of AI applications will be applied, and any potential risks associated with sharing their data.
However, in the context of AI, informed consent can be challenging, as patients may not fully understand the complexities of machine learning models and their implications. Healthcare providers must ensure that consent forms are written in clear, accessible language, and that patients are provided with the necessary information to make informed decisions.
Moreover, ongoing consent management is crucial in the evolving landscape of healthcare AI. Patients should have the right to revoke consent or modify their preferences at any time. Healthcare organizations and AI developers must establish mechanisms for managing and respecting these changes to consent in real-time.
7. Addressing Bias and Fairness in AI Models
Data privacy is closely linked to the ethical use of AI, which includes addressing concerns related to bias and fairness. AI models trained on biased or incomplete data can perpetuate disparities in healthcare outcomes, disproportionately affecting certain patient groups. This could lead to privacy violations, particularly if certain patient populations are systematically excluded from the benefits of AI-driven healthcare advancements.
Healthcare AI applications must be designed to ensure fairness and equity. This includes ensuring that datasets used for training are diverse, representative, and free from discriminatory biases. Regular audits of AI models should be conducted to ensure that they are not inadvertently reinforcing existing healthcare disparities.